Generative Artificial Intelligence (AI) represents a fascinating and rapidly evolving frontier in the field of technology. It encompasses systems and models capable of creating new content, whether it's text, images, music, or even entire virtual worlds. This article provides an overview of generative AI, explaining what it is, how it works, and its various applications.

What is Generative AI?
Generative AI refers to a class of AI systems designed to generate new content by learning patterns and structures from existing data. Unlike traditional AI, which primarily focuses on analyzing and interpreting data, generative AI actively creates. This capability has profound implications across numerous industries, from entertainment to healthcare.
How Does Generative AI Work?
Generative AI relies on advanced machine learning models, particularly those within the domain of deep learning. Here are the key components and mechanisms that make generative AI possible:
1. Neural Networks
Neural networks are the backbone of generative AI. These networks are inspired by the human brain's structure and function, consisting of layers of interconnected nodes (neurons) that process and learn from data.
2. Training Data
Generative AI models require vast amounts of training data. This data serves as the foundation for learning the patterns and nuances needed to generate new content. For instance, a generative AI model trained on thousands of images can learn to create realistic-looking pictures.
3. Algorithms and Architectures
Several algorithms and architectures are pivotal in generative AI, including:
Generative Adversarial Networks (GANs): Introduced by Ian Goodfellow in 2014, GANs consist of two neural networks, the generator and the discriminator, which are pitted against each other. The generator creates content, while the discriminator evaluates it. Through this adversarial process, the generator improves over time, producing increasingly realistic outputs.
Variational Autoencoders (VAEs): VAEs encode input data into a compressed representation and then decode it back to generate new content. They are particularly useful for tasks requiring smooth and continuous content generation, such as generating variations of images.
Transformers: Initially developed for natural language processing, transformer models like GPT-4 (Generative Pre-trained Transformer 4) have revolutionized text generation. These models leverage self-attention mechanisms to understand context and generate coherent and contextually relevant text.
4. Fine-Tuning and Optimization
After initial training, generative AI models often undergo fine-tuning. This process involves adjusting the model's parameters using specific datasets to enhance its performance in particular applications. Optimization techniques, such as gradient descent, are employed to minimize errors and improve output quality.
Applications of Generative AI
Generative AI's ability to create new content has led to a plethora of innovative applications across various fields:
1. Content Creation
In the media and entertainment industry, generative AI is used to create music, art, and even scripts. For example, AI-generated music can provide soundtracks for games and movies, while AI-generated art can inspire new forms of digital creativity.
2. Natural Language Processing
Generative AI powers advanced language models capable of writing articles, generating poetry, and even engaging in human-like conversations. Chatbots and virtual assistants leverage these models to provide more natural and coherent interactions.
3. Healthcare
In healthcare, generative AI aids in drug discovery and medical imaging. By generating molecular structures, AI can help identify potential new drugs. Similarly, in medical imaging, AI can create detailed images to assist in diagnosis and treatment planning.
4. Gaming and Virtual Reality
Generative AI is transforming the gaming industry by creating immersive environments, characters, and narratives. It enables dynamic content generation, making games more engaging and personalized for players.
5. Data Augmentation
In machine learning, generative AI is used for data augmentation, creating synthetic data to enhance training datasets. This is particularly valuable in scenarios where acquiring large amounts of real-world data is challenging or expensive.
Challenges and Ethical Considerations
Despite its potential, generative AI also presents challenges and ethical considerations. Issues such as copyright infringement, the creation of deepfakes, and the potential for biased or harmful content generation require careful regulation and oversight. Ensuring the responsible use of generative AI is paramount to maximizing its benefits while mitigating risks.
Conclusion
Generative AI stands at the forefront of technological innovation, offering transformative capabilities across a multitude of domains. By understanding what generative AI is and how it works, we can better appreciate its potential and navigate the ethical landscape it presents. As the field continues to evolve, generative AI promises to unlock new realms of creativity and problem-solving, shaping the future in unprecedented ways.
Join the AI Tech Event in Delhi on July 5, 2024, to explore more about Generative AI, where eminent speakers will share their knowledge.
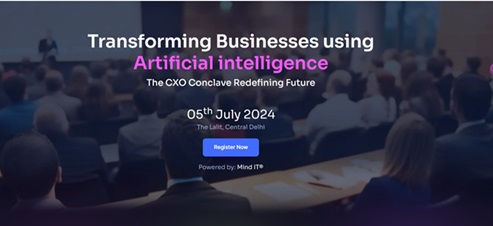
Comments